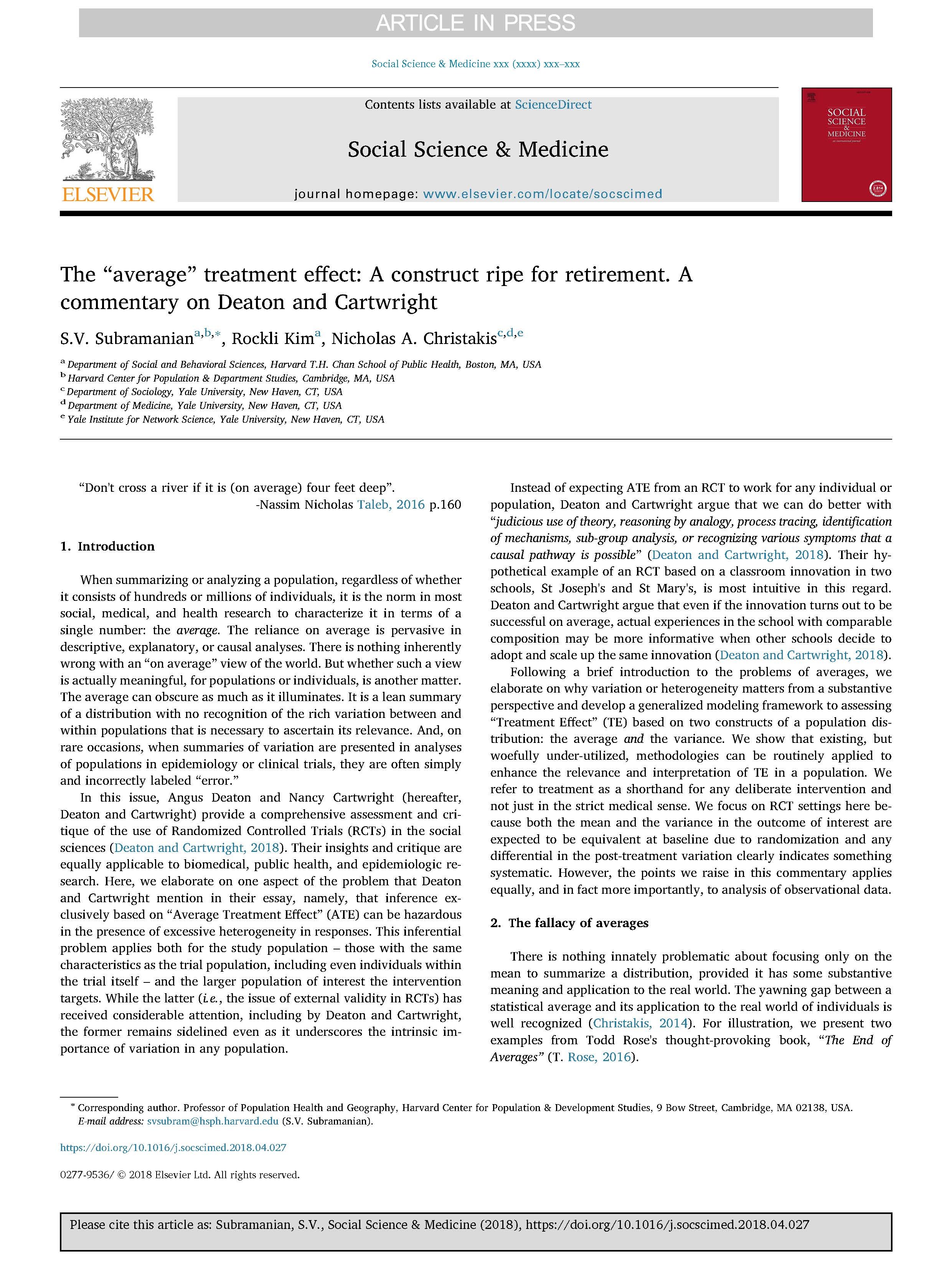
The “average” treatment effect: A construct ripe for retirement. A commentary on Deaton and Cartwright
Abstract
When summarizing or analyzing a population, regardless of whether it consists of hundreds or millions of individuals, it is the norm in most social, medical, and health research to characterize it in terms of a single number: the average. The reliance on average is pervasive in descriptive, explanatory, or causal analyses. There is nothing inherently wrong with an “on average” view of the world. But whether such a view is actually meaningful, for populations or individuals, is another matter. The average can obscure as much as it illuminates. It is a lean summary of a distribution with no recognition of the rich variation between and within populations that is necessary to ascertain its relevance. And, on rare occasions, when summaries of variation are presented in analyses of populations in epidemiology or clinical trials, they are often simply and incorrectly labeled “error.”
In this issue, Angus Deaton and Nancy Cartwright (hereafter, Deaton and Cartwright) provide a comprehensive assessment and critique of the use of Randomized Controlled Trials (RCTs) in the social sciences (Deaton and Cartwright, 2018). Their insights and critique are equally applicable to biomedical, public health, and epidemiologic research. Here, we elaborate on one aspect of the problem that Deaton and Cartwright mention in their essay, namely, that inference exclusively based on “Average Treatment Effect” (ATE) can be hazardous in the presence of excessive heterogeneity in responses. This inferential problem applies both for the study population – those with the same characteristics as the trial population, including even individuals within the trial itself – and the larger population of interest the intervention targets. While the latter (i.e., the issue of external validity in RCTs) has received considerable attention, including by Deaton and Cartwright, the former underscores the intrinsic importance of variation in any population and remains sidelined.
Instead of expecting ATE from an RCT to work for any individual or population, Deaton and Cartwright argue that we can do better with “judicious use of theory, reasoning by analogy, process tracing, identification of mechanisms, sub-group analysis, or recognizing various symptoms that a causal pathway is possible” ( Deaton and Cartwright, 2018, p. XX). Their hypothetical example of an RCT based on a classroom innovation in two schools, St Joseph's and St Mary's, is most intuitive in this regard. Deaton and Cartwright argue that even if the innovation turns out to be successful on average, actual experiences in the school with comparable composition may be more informative when other schools decide to adopt and scale up the same innovation (Deaton and Cartwright, 2018).
Following a brief introduction to the problems of averages, we elaborate on why variation or heterogeneity matters from a substantive perspective and develop a generalized modeling framework to assessing “Treatment Effect” (TE) based on two constructs of a population distribution: the average and the variance. We show that existing, but woefully under-utilized, methodologies can be routinely applied to enhance the relevance and interpretation of TE in a population. We refer to treatment as shorthand for any deliberate intervention and not just in the strict medical sense. We deliberately focus on RCT settings here because both the mean and the variance are expected to be equivalent at baseline due to randomization and any differential in the post-treatment variation clearly indicates something systematic. However, the points we raise in this article applies equally and in fact more importantly, to analysis of observation data.
Citation:
S.V. Subramaniana, Rockli Kima, Nicholas A. Christakis, "The “average” treatment effect: A construct ripe for retirement. A commentary on Deaton and Cartwright" Social Science & Medicine,